Introduction
Global Positioning System (GPS) have been usually used to study animal behavior in both range systems and dary farm, mainly to quantify positions and movements of cattle and others ungulate species, and derive measurements about their grazing and resting activities (Black et ál. 2008, Depew 2004, Ganskopp 2001). On the other hand, there is a long history of ecologists and environmental scientists using radio-transceivers and position data from the GPS to track and monitor the behavioral ecology of free ranging animals (Tomkins & O›Reagain 2007). Also, Handcock et ál. (2009) say that cattle behavior monitoring data have been used to explore a number of concepts around correlating GPS data and satellite remote-sensing data for improved information about herd behavior as a function of the animals’ environment, they have presented a conceptual overview and discussion around combining ground-based sensing and remotesensing observations within a Wireless Sensor Networks (WSN) to derive improved information about animal behavior in the environment. Use of WSN for automated livestock monitoring and control is a technology with significant potential in the immediate future to study animal behavior. Sometimes, it is difficult to achieve practical and reliable cattle monitoring with current conventional technologies due to challenges such as large grazing areas of cattle, long time periods of data sampling, and constantly varying physical environments (Guo et ál. 2006). This network bring a new level of possibilities into this area with the potential for greatly increased spatial and temporal resolution of measurement data.
Some studies suggest important inconsistencies in the measurements using GPS, therefore it is important to carry out basics inquiry for clarify the limitations of these methods. There still is uncertainty in the accuracy of individual locations, however, a 95% confidence interval of about 100 m is more precise than other telemetry methods (Moen et ál. 1996). In addition, with the implementation of differential correction in data from GPS collars, accuracy will increase by about an order of magnitude, with an expected 95% confidence interval of about 12 m (Moen et ál. 1996) and others studies found a radius of 5.5 m from the mean locale, encompasses 90% of the coordinates, they refer an anomaly of the Geographic Positioning System is that more error occurs for north-south or Y-axis values than among east-west or X-axis determinations (Gasknoop & Jonhson 2007).
Complete accuracy in activity determinations is difficult to achieve for several reasons: 1) there is an indirect connection between the independent and dependent variables, and there are many potential sources of noise in the data. 2) Resting activity is not always associated with zero or very low motion sensor counts using Lotek GPS collars (Ungar et ál. 2005). About it, Ganskoop & Jonshon (2007) suggest that GPS error between a series of coordinates always generates a positive value that, in applied studies, may mistakenly be perceived as travel, although GPS collar have been stationary. The bulk of perceived movements, therefore, likely accumulate when collared animals are inactive and can inflate estimates of daily travel by cattle as much as 15%.
So, collar application parameters and performance characteristics have undergone preliminary investigation. Standardized collar mounting and calibration procedures will need documentation, allowing for effective data collection from future experiments in this research area (Turner et ál. 2000). For example, the effect of pasture size upon GPS collaring technology should be investigated. Cattle in small, familiar, intensively managed paddocks may exhibit different herd behavior than those in extensive rangeland gra- zing situations. Extensive grazing systems may require different cow collaring protocols. Co- llared dominant or social cows may represent herd location adequately and herd behavior may be estimated. Fenceless paddocks employing “real time” GPS systems to guide animal behavior pose an exciting opportunity. Monitoring livestock with GPS technology offers meaningful data from which research-based results can be obtained to improve such efforts (Turner et ál. 2000).
Our goal of this article is contribute to confirm the usefully and limitations of the studies using GPS collars and show the importance of the empirical watching of animal activities, in order to improve the confidentiality of measurements.
Materials and methods
The study was conducted at the robotic and pasture-based dairy farm of the WK Kellogg Biological Station (42o24’57’’ N; 85o22’58’’ W), Michigan State University, USA, between August 11 and 20 of 2010. The farm has 75 ha of irrigated pasture divided in paddocks of approximately 2 to 8 ha. We used six paddocks of two ha each, where the cows were grazing during 6 daylight.
Initial tests were conducted at testing accuracy of GPS. Two Lotek collar was placed vertically about 240 hours to 10 days in paddocks nearby the experimental pastures, at 1.65 m above ground. Geographic Coordinates of those GPS Lotek was registered using a high accuracy submetric GPS (Topcon GMS-2). In late August we downloaded the data and the locations from the GPS unit were differentially corrected. Our goal of this test was determine the accuracy of the data getting with Lotek GPS.
Visual scans of foraging activities and recording of locations and activity sensors was conducted on 4 lactating Holstein dairy cows (650 kg LW; 23 kg.day-1) equipped with GPS collars (Lotek Inc., city, Canada) containing head position, X-axis and Y-axis movement sensors. In addition, this collars allows to determine animal behavior as a function of ambient temperature using a temperature sensor. Foraging activity scans were conducted at 5 minute intervals by 4 trained observers during 6 daylight periods of 4 hours. The target-cows were companioned with 40 dairy cows grazing (forage availability > 20000 kg.ha-1 DM). Scanned activities were grazing, traveling, standing, standing rumina- ting, lying, and lying ruminating. Post-processing activities as lying, lying ruminating, standing ruminating and standing activities were pooled like resting. Finally, we worked with three cate- gories: traveling, grazing and resting.
Observers were able to maintain a distance of 10 to 50 m of focal cows without any noticeable change in behavior. Following watches were synchronized with the time of GPS’s collars installed in cows, in order to record activities simultaneously.
Total distance walked and distance between consecutive GPS fixes was derived from differentially corrected GPS data using ArcGIS 9.3. Thus, following to Ungar et ál.(2005), using GPS units in conjunction with Geographic Information Systems (GIS), grazing distribution and animal movement was related to landscape features.
Sensor activity and distance between fixes was compared to visual scans of foraging activities using statistical package Infostat (2010). For each of the four cow 12 hours average per cow were registered, for a total amount 2830 hours.
Results and discussion
Results from GPS collars showed that there was 82-86% probability of estimating animal locations with a 7 m error. That range of distance was obtained from reference GPS represented as green points (Figure 1). Ganskopp and Jonhson (2007) found a radius of 5.5 m from the mean locale (X), encompasses 90% of thecoordinates. An anomaly of the Geographic Positioning System is that more error occurs for north south or Y-axis values than among east-west or X-axis determinations.
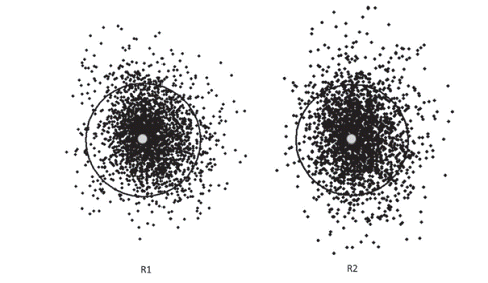
Fig. 1 The circle depicting a radius of 7 m from the reference point (R) encompasses 86% (R1) and 82% (R2). Diamonds represent GPS logs every 5 minutes.
In terms of Ganskopp and Johnson (2007) given the random nature of GPS error measures of distance between adjacent GPS coordinates for moving collars could be remarkably accurate with a low bias for distances between 10 to 90 m, but, when a GPS collar is stationary, however, GPS error between a series of coordinates always that generates a positive value, in applied studies, may mistakenly be perceived as travel. Thus, precisely why this test is important for our study because it quantifies what is the threshold that discriminates activity no activity.
Time cattle devoted to specific activities
GPS data suggested the cows were on pasture the most part of time 94.6% (±0.92) and under barn only 5.4% (±0.92). When the cows were on pasture, the most time they spent grazing (51%); other part of the time was devoted to resting (43%) and traveling (6%); cattle traveled an average total 3385 m ±712 SE per day, however, Johnson et ál. (2009) showed that daily travel distance was about 5 170 m day-1 in Oregon, USA.
Probably, the most proportion of grazing has been affected by the monitoring system carrying out mainly during daylight periods (from 5 pm to 9 pm). On the contrary, under barn, the cows were mainly resting (87.1%), less time spent them in feeding and drinking (9.9%) and traveling (3.1%) activities. In total (grazing + barn), we can infer that during 24 hours, the animals were 49% (12 h) grazing, 5.8% (1 h) resting and 45% (11h) traveling.
Our data are very far from studies of daily activity with Charolais cattle of 6.1 h / day grazing and 9.2 h.day-1 resting (Gary et ál. 1970). Probably, high production dairy cows may have extended grazing time as an attempt to increase forage intake and compensate high milk production requirements.
A study in Oregon suggested cattle rested (standing or bedded) for 10.1 h and foraged for 11.0 h each day. The remaining 2.9 h were divided among walking (1.8 h), drinking (0.3 h), and mineral consumption (0.3 h) (Ganskopp 2001). In our case, probably, due the high yield of dairy cattle spend more time grazing, because they need intake dry matter to fill requirements to keep high milk production.
A first set of data recorded by the GPS were analyzed to compare the activities of the cows and their corresponding distances recorded by these devices. Empirical distribution of values from distance traveled by cows was plotted (Figure 2) for each cattle-GPS collars. We visually identified the threshold of the curve as at each activity for separating resting and active periods (Figure 2). Thus, added cutting lines, you can easily identify the 75% percentile that It corresponds to the value 9 (x axe), which suggests that no activity (resting) is characterized by smaller distances between positions of 9 m.
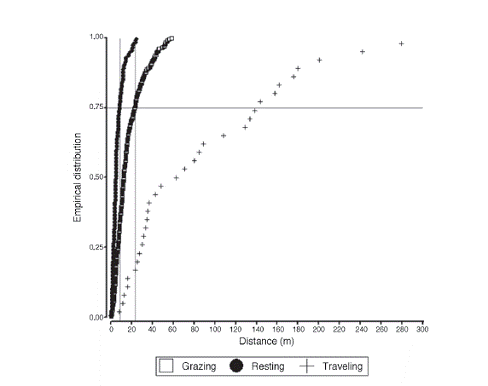
Fig. 2 Empirical distribution to activities in terms of fixes (each 5-min) observation: G, grazing; T, traveling and R, resting.
The box-plot, is intended to better reflect the shape of the frequency distribution, resulting in one graph about the median, average, quartiles 0.05, 0.25, 0.75 and 0.95 and showing the presence, if any, outlier (Di Rienzo et ál. 2008). Therefore, using the third quartile (0,75; upper limit of the box) as upper class limit of the distance (m) registered by GPS, we discriminate the Resting, Grazing and Traveling activities of the cows, in order to discriminate classes of observed activities, thus, we discriminate three classes: <8.6 m, resting; 8.6-23.4 m, grazing, and > 23.4 m, traveling (Figure 3). Those class limits were used to classify distances registered with GPS along complete day. After that we compare observed activities with predicted activities.
Association between observed data and predicted data
An analysis between observed fixes and predicted data show that 60% were coincident in the observations of Non Activity (lying and standing). But, in observations of Activity (Grazing, traveling and standing) the observed data and predicted data show that 80% were coincident. These data were statistically analyzed with Chi-square test, shown a dependence between observed and predicted data (p=0.05).
A similar study was carried out in Colombia Using the third quartile as up class limit of the distance (m) registered by GPS in each activity (Resting, Grazing and Traveling) of cows, defined classes of observed activities. Thus, they established three classes (<4 m, resting; 4-12 m, gracing; and > 12 m, traveling); their analysis between observed positions and predicted data show that 72% were coincident in the observations of No activity (resting) with Chi-square test, shown a dependence between observed and predicted data (p=0.001) (Mora et ál. 2014).
Data of X-act and Y-act sensors are confuses in our studies, however, previous research using GPS collars to monitoring cattle behavior found left-right motion sensor counts best correlated with foraging and walking activities of animals (Ungar et ál. 2005, Ganskopp & Bohnert 2006).
We found that the data of temperature sensor was instructive to classify activity under different temperature ranges (Low <25ºC; Medium 25-30ºC and High >30ºC). Under Low temperature the main activity of cows was grazing (92%) and under Medium and High temperature grazing was only 62.6 and 59.4%, respectively. On the contrary, resting was important under Medium and High temperature (33.6 and 31.8%, respectively) (Figure 4).
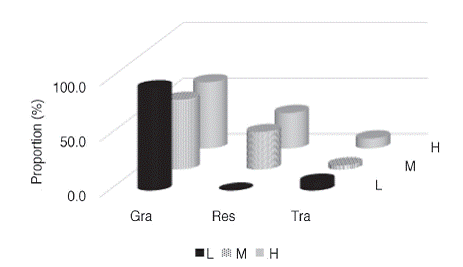
Fig. 4 Relations between sensor temperature classes registered by Lotek GPS and proportion of activities on the pasture.Temperature classes: Low (L), medium (M), high (H).Activities: Grazing (Gra); Resting (Res); Traveling (Tra).
A study carried out in Colombia (Serrano 2013) showed that generally, cows prefer to devote more time resting under higher temperatures and less to traveling. Particularly the F1 cows, during the warm period, devoted more time traveling, on the contrary, at the hot period, they spent more time at grazing or resting. The Brahman cows, on warm period, spent more time traveling in comparison to the hot period. This behavior is relating to thermoregulation livestock process, because they are associated to expend additional energy to maintain body temperature when temperatures are above or below the zone of thermoneutrality and seek out shelter to avoid extremes of heat and cold (National Research Council 1996).
Conclusions
The use of Global Positioning Systems (GPS) to discriminate foraging activities of dairy cattle grazing temperate pasture was evaluated and the usefulness of this device was demonstrated to monitor animal behavior.
Data suggested the cows were on pasture the most part of time. When the cows were on pasture, the most time they spent grazing; other part of the time was devoted to resting and traveling. Probably, the most proportion of grazing has been affected by the monitoring system carrying out mainly during daylight periods.
We propose that is necessary to make adjustments and calibrations to improve the metho- dology in order to reduce the GPS data bias.