Introduction
Gorse (Ulex europaeus, family Fabaceae) is a spiny, perennial, evergreen shrub native to Central and Western Europe, where seasonal weather changes and several pathogens and herbivores limit its prevalence. This plant is known for its highly competitive nature, characterized by two main features. Firstly, it forms dense structures effective at blocking out and displacing other native and cultivated species. This is bolstered by its mycorrhizal connections that help support its growth even in nutrient-poor soil by fixing nitrogen (Egunjobi, 1969; Grubb & Suter, 1970; Invasive Species Specialist Group, 2022; Vargas, 2007) Secondly, gorse has a high seed-production rate that leads to a density ranging from 500 to 20 742 seeds/m2 (Ivens, 1978; Popay & Adams, 1990). These seeds boast an impressive viability rate of over 80 % and can remain viable in the soil seed bank for up to 30 years (Lee et al., 1986; Hoshovsky, 1989). Barochory dispersal plays an important role in taking seeds up to 6 m from the plant, which can be greater due to wind (Moss, 1959). Additionally, gorse quickly matures and grows up to 0.5 m per year under ideal conditions (Lee et al., 1986).
Out of its native range, the consistent weather patterns allow gorse to flower year-round, and the lack of predators places no natural limit on its spread. This adaptability has allowed gorse to successfully colonize Andean high-mountain tropical ecosystems, even at altitudes exceeding 2 000 meters above sea level, where temperature and precipitation closely resemble its native habitat during the flowering season (Zabaleta-Bejarano, 2008). However, gorse is a dangerous invasive species for the Andean ecosystems since it i) contains highly flammable oil in its foliage and seeds and ii) accumulates standing necromass, which together represents an extreme fire hazard that yields hotter fires than other weeds (MacCarter & Gaynor, 1981). For instance, gorse has been included in the top 10 invasive species of concern with a rating of 7.39 out of 10 in the Risk Assessment of Introduced Plant Species in Colombia report, which means a high risk of invasion (Baptiste et al., 2010; Calderon, 2003).
Indeed, gorse represents a significant threat to Colombia’s exceptional biological richness. This country hosts approximately 10 % of the world’s biodiversity and has around 2 % of its land covered by paramos (Convention on Biological Diversity, n.d.), a vital ecosystem located over 2 800 meters above sea level. The Andean paramo is considered the most biodiverse high-mountain tropical region globally and one of the most important ecosystems for human well-being. Nonetheless, this delicate ecosystem is highly vulnerable to invasive species like gorse, which can disrupt its natural balance and have far-reaching impacts on millions of people dependent on essential ecosystem services, such as drinking water (Anthelme & Peyre, 2019; Buytaert et al., 2006; Sklenar et al., 2014).
To address this issue, it becomes imperative to undertake thorough assessments of the extent of gorse invasions in the region and quantify its biomass to develop effective eradication programs. Currently, some models allow for biomass estimations based only on the plant’s height, meaning no destructive techniques (e.g., burning) are required. For example, Pearce et al. (2010) developed linear mixed-effect models to estimate aboveground biomass (AGB) and aboveground available fuel (hereafter fuel load) in gorse shrublands by collecting samples from most of the climatic zones in New Zealand.
With the rapid expansion of UAS (Uncrewed Aircraft Systems) remote sensing methods, estimating AGB at a low cost has become possible. These methods accurately estimate canopy height (Acorse et al., 2019) and aboveground volume, which are essential to derive AGB (Peng et al., 2021). In some cases, only optical images (RGB) are necessary to estimate AGB, as demonstrated by Niu et al. (2019), who quantified AGB from vegetation indices and plant height datasets derived from UAS-based RGB imagery. The same methodology can be applied to fuel load quantification as the latter depends on the same variables. Despite these recent advancements, there are no guidelines for gorse AGB estimation in Colombia. The Institute of Hydrology, Meteorology, and Environmental Studies (IDEAM in Spanish) has released a general protocol for AGB and carbon estimation for non-tree vegetation, which includes shrubs, but through destructive sampling (Yepes et al., 2011). The literature about UAS-based imagery for AGB in Colombia is also scarce or null. Only a few studies have quantified AGB nationally using low-resolution satellite imagery, such as MODIS (Anaya et al., 2009; Galindo et al., 2011).
It is crucial then to identify the places that gorse has colonized, quantify the area affected by the invasion, and estimate its biomass to establish adequate, sustainable environmental management strategies oriented to eradicating the species, as this is a threat to Colombia’s native biological resources. Therefore, the overarching goal of this study is to estimate gorse AGB and fuel load within a paramo neighboring Los Nevados National Natural Park (Tolima, Colombia) using remote sensing imagery. First, an object-based supervised classification quantifies the total area invaded using i) high-resolution UAS-based RGB imagery and ii) a Normalized Difference Vegetation Index (NDVI) derived from Planet imagery. Then, the allometric equations Pearce et al. (2010) reported are used to estimate gorse AGB and fuel load. The methods applied here can be extended to other areas invaded in Colombia and beyond to propose large-scale ecological management strategies of gorse.
Likewise, this study aims to provide preliminary results and context which will be valuable for further multidisciplinary research projects to build on to propose a comprehensive program for gorse management. This may be a bioeconomic plan for the industrial use of gorse biomass that also promotes the local economy and industry. Bioeconomy, as defined by the Organization for Economic Cooperation and Development (OECD, 2009), encompasses the use of biological resources for economic activities oriented to boost industrial processes and enhance environmental sustainability. For instance, Miller & Murthy (2017) found that 30.2 ± 5.8 gallons of ethanol can be extracted from one ton of gorse. This information can be used to treat gorse as a biological source of ethanol (bioethanol) and encourage eradication programs. Hence, this study uses the AGB results to quantify the potential bioethanol production generated from exploiting gorse. In addition, bioethanol market prices are also consulted to assess the potential economic benefits for the local community and industry.
Materials and methods
Study area: Situated within the Lagunilla River valley, the study area is positioned at 4°53’32” N & 75°15’20” W, with an elevation exceeding 3 250 meters above sea level in the department of Tolima, Colombia. The deforestation due to the expansion of the farming frontier, the edge effect caused by a road and the river, and the presence of poor soils have favored gorse colonization (Fig. 1). This species here is problematic as this area is within the paramo zone neighboring Los Nevados National Natural Park: a protected area that supports the economic and human development of the country’s central region (Parques Naturales Nacionales de Colombia, n.d.).
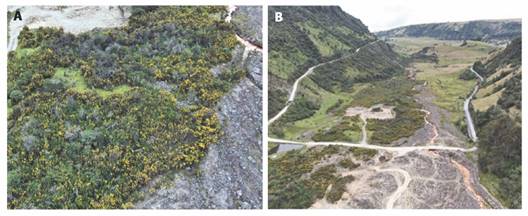
Fig. 1 Invasion of Gorse in the Study Area (Source: Cortolima, personal communication, 2022). A. A close-up photo depicting gorse, characterized by its standing necromass and yellow flowers, suffocating native paramo species (non-flowered vegetation). B. A long shot showing the extensive invasion of gorse in the flat valley landscape, marked by a mixture of green and yellow vegetation.
Supervised classification: The raw UAS-based RGB imagery (hereafter UAS imagery) was provided by the Regional Autonomous Corporation of the Department of Tolima (Cortolima). The dataset consisted of 439 images taken on July 10, 2022, covering a total area of 0.664 km2. They used a camera model FC6520 lens DJI MFT 15mm F1.7 ASPH with a photo resolution of 5280 x 2970. The imagery was processed in Pix4Dmapper version 4.7.5. All images’ horizontal and vertical accuracy was set at 1 m, and the coordinate system WGS 84 UTM zone 18N (EGM96Geoid) was selected as the spatial reference. An orthomosaic with 12.51 cm pixel resolution and an unsigned 8-bit pixel depth was obtained.
Also, a Digital Surface Model (DSM) was generated using the default method of inverse distance weighting (IDW), and a Digital Terrain Model (DTM) was produced to subtract its values from the DSM to get a normalized DSM (nDSM). Pix4D allows for deriving a high-quality DTM using an algorithm based on the semiautomatic method developed by Unger et al. (2009) that smoothens the DSM using a variational energy minimization approach. To improve the DTM accuracy, manual polygons were created over the point cloud to specify roads, ground, and high-vegetation classes, so that only the latter class was smoothened.
Due to the lack of a near-infrared (NIR) band in the UAS imagery, Planet imagery including NIR was also obtained: ortho scene with catalog ID 20220808_151753_40_2274 from strip 5842545 taken on August 8, 2022 (less than one month after the UAS imagery). This image was radiometrically-, geometrically-, and sensor-corrected by Planet before downloading using the cubic convolution resampling algorithm. It also had a 3 m pixel size (after orthorectification), an unsigned 16-bit pixel depth, and a WGS 84 UTM zone 18N map projection (Planet, 2017).
In ArcGIS Pro version 3.0.2, a polygon shapefile was manually created to represent the area of interest (AOI), defined as the zone where gorse was visibly identified within the study area (Fig. 2). The UAS and Planet orthophotos were clipped to this area. To enhance gorse identification, the Raster Calculator tool was used to i) generate the normalized difference vegetation index (NDVI) from Planet NIR and red bands and ii) obtain the nDSM. Subsequently, the UAS orthophoto, the NDVI, and the nDSM were incorporated into a new project using eCognition version 10.2. This software was chosen as it has been demonstrated to have highly effective object-based image analysis (OBIA) methods for producing accurate classification maps of invasive plants (Michez et al., 2016; Lourenco et al., 2021).
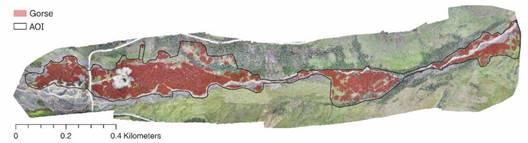
Fig. 2 Predicted distribution of gorse across the AOI within the study area. Imagery from Cortolima (personal communication, 2022).
All the parameters used in the eCognition ruleset were determined through trial and error. First, the ruleset consisted of a multi-threshold segmentation using the nDSM. A lower threshold of 0.5 m was set to exclude most of the ground, while an upper threshold of 6.5 m was determined through manual inspection, as no gorse plants were found to exceed this height. Any pixel values out of this range were classified as Other, a class of no interest, while those within the range were left unclassified. Then, a multi-resolution segmentation was applied to the unclassified class using a scale parameter of 20, a shape of 0.1, and a compactness of 0.5. To remove most of the non-gorse areas, everything with an NDVI value less than 0.54 was classified as Non-vegetated. Similarly, the texture was used to mask out other types of vegetation. The GLCM Homogeneity (quick 8/11) All Directions algorithm with the UAS red and green bands was used to classify as Other all the objects with values greater than 0.06 and 0.048, respectively. For the UAS blue band, the GLCM Mean (quick 8/11) All Directions algorithm was used to remove objects with values greater than 130.
Training sample polygons were created in ArcGIS Pro and added to the eCognition project for supervised classification. To ensure accurate classification, a temporal class was created to classify one-pixel-size objects, which are problematic for texture analysis, and merge them into the surrounding classes. The Random Forest machine learning algorithm was chosen as it is one of the most accurate classifiers (Fernandez-Delgado et al., 2014). The parameters used were: 30 for depth, 0 for minimum sample count, 16 for maximum categories, and 500 for maximum tree number. This number of trees was selected since it has a low influence on the model execution (Granzig et al., 2021).
The objects with the classification and the mean nDSM were exported to separate shapefiles. The misclassified objects were then reviewed and corrected by generating a 150 m cell-size grid using the Create Fishnet tool in ArcGIS Pro. Each cell was visually inspected at a 1:300 scale, and the classification was approved if the visible error was within the acceptable range of 15-20 %, as suggested by Peyre et al. (2021). These acceptable errors referred to mismatches between non-flowered gorse and non-gorse vegetation. Next, only the gorse class was exported to a new shapefile.
To assess the classification accuracy, first, the Non-vegetated and Other classes were grouped into a single class called No-gorse. Then, a stratified random sampling approach was employed using the Create Accuracy Assessment Points tool in ArcGIS Pro. A total of 245 samples were selected according to the equation developed by Cochran (1977):
where n is the number of samples, Z is the confidence interval (set at 95 % or 1.96), P is the expected overall accuracy (set at 0.8), and E is the allowed error (set at 0.05). Later, a new field was created in the attribute table of the points to add ground reference values. To avoid bias, the “Classified” field in the attribute table was hidden during the ground reference data collection process. As no other data were available, the ground reference values were derived from the same UAS orthophoto used for the classification. Finally, a confusion matrix was generated to calculate various accuracy metrics, including overall accuracy and the Kappa coefficient.
AGB, fuel load, and bioethanol quantification: First, Pearce et al. (2010) developed a pair of linear equations to estimate gorse AGB and fuel load:
ln(AGB) = 1.44 + 0.872 * ln(OsHt) + ln(1.02)
ln(fuel load) = 0.848 + 0.552 * ln(OsHt) + ln(1.04)
where OsHt is overstorey log height (m), and the ln() at the end is a correction factor for the bias introduced when exponentiating the functions to obtain AGB and fuel load (kg/m2) in dry weight basis (db). According to the authors, the equations yield an average precision of 30 % for AGB and 40 % for fuel load. For this study, the equations were inserted in the ArcGIS Pro Calculate Field tool, taking the objects’ mean nDSM values as the overstorey log height, which generated AGB and fuel load density values for each object. Next, the densities were multiplied by the area of each object to obtain the mass values (kg), and the total AGB and fuel load over the whole AOI were obtained by summing the values for all the objects.
Second, Miller & Murthy (2017) found that 30.2 ± 5.8 gallons of ethanol can be extracted from one ton of gorse (db), increasing to 30-40 % more if C5-sugar fermenting microorganisms are utilized besides yeast. These values were used with the AGB calculated above to estimate the potential gorse bioethanol content in the AOI. In addition, the potential income from bioethanol production was calculated based on information provided by the National Federation of Biofuels of Colombia about the ethanol market price during the second semester of 2022, which corresponded to COP 13 048/gal. To convert this price to USD, the average annual exchange rate for 2022 was used, which was USD 1 = COP 4 257.68. Consequently, the calculations’ unit price was USD 3.06/gal of bioethanol.
Results
The total area invaded is estimated to be 66 465 m2, representing around 10 % of the study area (as illustrated in Fig. 2), where the average gorse height is 2.05 m.
The findings of the accuracy assessment demonstrate an overall accuracy rate of 85.3 %. Notably, the producer’s accuracy for the gorse class was observed to be relatively low when contrasted with that of the no-gorse class. This discrepancy suggests a greater incidence of omission error in the classification of the gorse class. Specifically, the analysis identified that 33 points, which were associated with the gorse class, were misclassified as not being gorse, whereas, in the case of the no-gorse class, only three points were inaccurately classified as gorse. Conversely, the user’s accuracy for the gorse class demonstrated a high value, indicating a low commission error, as 83 out of 86 points were correctly predicted as gorse. In addition, a Kappa coefficient of 70.1 % shows that the classification performed significantly better than what could be achieved by random chance, i.e., there is a substantial agreement between the two classes. Table 1 shows the results of the accuracy assessment.
Table 1 Confusion matrix of the supervised classification.
Mapped class | Reference | |||
Gorse | No-gorse | Row total | User’s Accuracy | |
Gorse | 83 | 3 | 86 | 0.965 |
No-gorse | 33 | 126 | 159 | 0.792 |
Column total | 116 | 129 | 245 | |
Producer’s Accuracy | 0.716 | 0.977 |
Overall accuracy = 85.3 %. Kappa = 70.1 %.
The results obtained for biomass considering the allometric equations average precision are i) AGB ± 30 % = 527 978.9 ± 158 393.7 kg, which yields an overall density of 7.94 ± 2.38 kg/m2 (when divided by the total area occupied by gorse); and ii) fuel load ± 40 % = 233 805.7 ± 93 522.3 kg, with a density of 3.52 ± 1.41 kg/m2. Likewise, Fig. 3 shows the estimation of the total potential bioethanol production and income generated based on the AGB ± 30 % results. Overall, the estimated bioethanol production ranges from 11 161.5 to 29 019.8 gals, depending on whether C5-sugar fermenting microorganisms are used and the percentage of the production increase. Thus, the generated income may vary from USD 34 205.3 up to USD 88 933.7.
Discussion
Gorse is a dangerous invasive species affecting Colombian paramos; therefore, it is crucial to seek a bioeconomic model encompassing environmental management strategies that lead to i) the eradication or species control processes and ii) generating economic benefits for local communities that are the most affected. Unfortunately, in Colombia, the quantification of AGB and fuel load is done through destructive practices that are labor-intensive and costly (Pearce et al., 2010; Yepes et al., 2011). Moreover, there is no easily accessible literature regarding UAS-based imagery for AGB quantification in the country, nor specific guides for gorse AGB and fuel load estimation. Hence, this study proposes a first approach to estimating gorse AGB and fuel load within a paramo zone neighboring Los Nevados Natural National Park using high-quality UAS-based RGB imagery and an NDVI layer derived from Planet imagery. Likewise, the study uses the AGB results to obtain initial estimations of the potential production of bioethanol, and income generated if all this gorse is exploited.
This project is novel in estimating gorse AGB and fuel load in Colombia using only remote sensing techniques. Both values obtained here align with the results from the studies cited by Pearce et al. (2010) to calibrate their models. Regarding gorse total biomass, Egunjobi (1969) reported a range between 0.68 and 10.86 kg/m2, including roots. As for fuel load, Anderson & Anderson (2009) found fuel load density ranging from 2.6 to 7.4 kg/m2. Basanta et al. (1988) and Soto et al. (1997) estimated similar values (2.5-6.0 kg/m2) for communities in the Atlantic. In Spain, Vega et al. (2005) obtained values from 4.6 to 5.2 kg/m2, which are slightly higher due to their focus on gorse shrubs measuring 2.3-2.4 m tall. Likewise, other research studies using the models by Pearce et al. (2010) and measuring gorse overstorey log height with UAS remote sensing methods have obtained similar results. Valencia et al. (2023a), Valencia et al. (2023b) found i) AGB and fuel load value ranges of 1.74-7.91 kg/m2 and 0.36-1.91 kg/m2, respectively, for populations whose average height was 1.1 m; and ii) a fuel load value ranging from 2.5 to 3 kg/m2 for populations around 1.5 m tall. Overall, the fuel load here represents an extreme fire hazard for the paramo ecosystem as nearly half of the gorse AGB is flammable.
As for the bioethanol production, the mean quantity obtained from gorse, 30.2 gal/ton, is higher than that extracted from conventional crops, such as sugarcane and sugar beets, which yield 19.5 gal/ton and 24.8 gal/ton, respectively (Shapouri & Salassi, 2006). This is because gorse has a high cellulosic content (Osorio-Castiblanco et al., 2019). Moreover, it is worth remembering that bioethanol extraction from gorse can increase by up to 40 % if C5-sugar fermenting microorganisms are used together with yeast. Under this scenario, the income generated ranges from around USD 48 000 to 89 000, which would be the first approach to attenuate the enormous economic cost caused by invasive species. Within Central and South America and the Caribbean Island region, Colombia ranks as the country with the second-highest average annual cost due to biological invasions: USD 191.77 million (Heringer et al., 2021). Furthermore, this money would benefit the local, low-income communities affected by the degradation of the ecosystem services they rely on. According to the National Administrative Department of Statistics (DANE, 2023) Tolima’s annual GDP per capita in 2022 was COP 22 253 352, or USD 5 226.64, when using the 2022 average annual exchange rate.
The accuracy assessment reveals that the model accurately predicts locations invaded by gorse. However, challenges arise in identifying all invaded locations, primarily due to the natural variability, and the different spectral signatures and textures of mature gorse plants. This issue is further compounded when dealing with green, non-flowered gorse plants, often leading to confusion with other vegetation. In parallel, a notable factor contributing to this complexity is the relatively large cell size of the NDVI layer (in comparison with the UAS orthophoto) which excluded some invaded areas along the river, road, and barren soil. Hence, the values obtained in this study may be slightly underestimated, meaning more gorse biomass may be present in the AOI, posing a higher risk to the paramo and Los Nevados National Natural Park.
The methodology developed for this study could be improved in several ways. For instance, LiDAR data would be beneficial to get ground returns where high, dense vegetation is predominant to improve the DTM and, thus, yield a more accurate nDSM, which is crucial to estimating log height for the allometric equations. A UAS-based NIR band is necessary to create a high-resolution NDVI layer to mask out the non-vegetated areas more accurately. Similarly, a deeper analysis of the ethanol content in gorse in the Andean region is recommended as the reference data used in the study is sourced from Oregon, US, and said content may vary geographically. Also, since gorse has a high lignocellulosic content, other bioeconomic projects may also be considered, such as carbon fibers from lignin, cellulose whiskers, etc. (Osorio-Castiblanco et al., 2019).
Finally, it is important to note that this paper only provides preliminary results about a bioeconomic model and, consequently, further research from multiple disciplines is needed to develop a successful, robust bioeconomic program leading to gorse eradication. Specifically, the program leaders must also consider the problems, challenges, and possible unintended consequences derived from the exploitation activities. These may include failing to affect gorse population growth; creating a highly economic, dependent market for locals who will be affected once the program is over; or promoting new invasions, among others (Nuñez et al., 2012).
In conclusion: Gorse (Ulex europaeus) has severely invaded an ecosystem within the paramo zone neighboring Los Nevados National Natural Park (Tolima, Colombia) and poses a high risk of further invasion and fire. This study is novel in Colombia as it represents the first attempt to identify the species and estimate its total aboveground biomass and fuel load over the affected ecosystem solely based on remote sensing data, primarily UAS-based imagery. Furthermore, the methodology outlined here can be extrapolated to other Andean ecosystems that have been invaded by gorse. Overall, the results showed AGB and fuel load densities of 7.94 ± 2.38 kg/m2 and 3.52 ± 1.41 kg/m2, respectively, which align with values reported in the literature. Since nearly half of the AGB corresponds to fuel load, urgent environmental management strategies are needed to control the exponentially growing expansion of the species and reduce the risk of fire. Additionally, this article proposes preliminary results on bioethanol extraction from gorse, which could be valuable for developing a comprehensive bioeconomic plan to encourage large-scale sustainable eradication programs and generate benefits for the surrounding low-income communities heavily reliant on the ecosystem services this paramo provides.
Ethical statement: the authors declare that they all agree with this publication and made significant contributions; that there is no conflict of interest of any kind; and that we followed all pertinent ethical and legal procedures and requirements. All financial sources are fully and clearly stated in the acknowledgments section. A signed document has been filed in the journal archives.