Introduction
Climate change, driven by anthropogenic actions, has the potential to modify the distribution patterns of biodiversity and the composition of ecological communities (Bellard et al., 2012; Kohler et al., 2010; Menéndez-Guerrero et al., 2020). Its effects include alterations in the spatio-temporal distribution patterns, increases in the probability of extinction, and declines in species richness (Clavel et al., 2011; Pounds et al., 2006; Stuart et al., 2004; Wake, 1991). Therefore, climate change could alter the beta diversity of anurans at the landscape level; declines in anuran species richness are projected, limiting ecosystem functions (Anderson et al., 2011; Magurran et al., 2019; Menéndez-Guerrero et al., 2020). Climate change favors the turnover of specialist species, with geographically restricted distribution patterns, by generalist species with wide spatial distributions, all without generating concomitant changes in species richness (Anderson et al., 2011; Bellard et al., 2012; Clavel et al., 2011; Magurran et al., 2019). Modeling the spatio-temporal distribution patterns of species with different reproductive modes and climate dependencies will help understand species-specific responses to global warming, acting as a framework for the development of conservation strategies.
The last glacial maximum (LGM) and the middle-Holocene (MH) are synonyms of climatic dynamics and environmental heterogeneity characterized by connection/disconnection processes between large tropical forests (Kohler et al., 2010; Sobral-Souza et al., 2015). These highly dynamic scenarios represented a challenge for anuran biodiversity that experienced variations in composition, abundance, and spatio-temporal distribution patterns (Pounds et al., 2006; Stuart et al., 2004; Wake, 1991). Although the tropical region exhibits high abundance, richness, and endemism of anurans, South America is the region where this taxon is most threatened (Fouquet et al., 2007; Menéndez-Guerrero et al., 2020; Stuart et al., 2004; Stuart et al., 2008). Specifically, the Neotropical region supports the greatest diversity of anurans in the world, with approximately 3 000 registered species (Frost, 2022). Anurans inhabit terrestrial and aquatic ecosystems, making this taxon particularly sensitive to the negative effects of climate change (Blaustein et al., 2010; Bruzzi-Lion et al., 2019; Medina et al., 2020; Menéndez-Guerrero et al., 2020). In fact, in recent decades Neotropical amphibian populations have decreased (Carey & Alexander, 2003; Clavel et al., 2011; Pounds et al., 2006; Stuart et al., 2004; Wake, 1991), with climate change being a determining factor on different spatio-temporal scales (Bellard et al., 2012; Menéndez-Guerrero et al., 2020).
Niche models calculate the n-dimensional hypervolume that defines the species’ ecological niches from the environmental dimensions of their conditions and resources (Hutchinson, 1957). Similarly, species distribution models (SDMs) allow inference of environmental niches in past, current, and future climate scenarios from the extrapolation of environmental predictors, and the occurrence records (Elith et al., 2006; Soberon, 2007). The family Leptodactylidae is a broad taxon and in Argentina is represented by 39 of the 232 species described (Frost, 2022). Leptodactylus latinasus (Jiménez de la Espada, 1875), has a wide geographic distribution including Argentina, Bolivia, Paraguay, Uruguay, and southern Brazil (Frost, 2022; Medina et al., 2020). Reproductive activity of L. latinasus begins with the rainy season (January-March) when temporary water bodies are still dry (Ponssa et al., 2019). Like many congeneric species, L. latinasus, lays its eggs in underground chambers within foam nests which prevent the larvae from drying out from desiccation (Downie & Smith, 2003; Ponssa & Barrionuevo, 2008). Larvaes hatch inside the chamber and reach bodies of water to complete their development (Bruzzi-Lion et al., 2019; Ponssa & Barrionuevo, 2008). Physalaemus cuqui (Lobo, 1993), is distributed in Bolivia, Paraguay, and Argentina (Iglesias & Natale, 2013). The reproductive activity of P. cuqui depends strictly on rain: this species presents a marked seasonality in oviposition, which occurs in floating foam nests inside temporary water bodies (Ferrari & Vaira, 2001).
Although the environmental and climatic requirements of both species are similar, the reproductive modes have contrasting ecophysiological constraints: How future climate change could modify the spatial distribution patterns of these species? We expect future climate change to decrease the environmental niche of both species, forcing the spatial distribution patterns to stable climatic latitudes (Bruzzi-Lion et al., 2019; Ferrari & Vaira, 2001; Medina et al., 2020). The objectives of this research were: 1) to determine the spatio-temporal distribution patterns of these sympatric species with contrasting reproductive modes and, 2) to evaluate whether the protected areas of the World Database on Protected Areas (WDPA), intended for the protection of biodiversity, allow the conservation of these species in the current scenario and in the future climatic changes scenario.
Materials and methods
Study site: We delimited the study area from the occurrence records of both species, restricted to South America (Fig. 1). The topographic landscape is represented by floodplains and highland ridges with air temperatures ranging between 20-26 ºC and annual rainfall between 2 200 and 3 100 mm, classified according to Köppen as Af and Am climates (Peel et al., 2007). The study area exhibits high spatial and environmental heterogeneity, incorporating different mosaics such as high-altitude mountain ranges, floodplains, mangroves, swamps, coastal regions, deserts, and savannahs interconnected by hydrographic basins and phytophysiognomic formations (Alvarez et al., 2022).
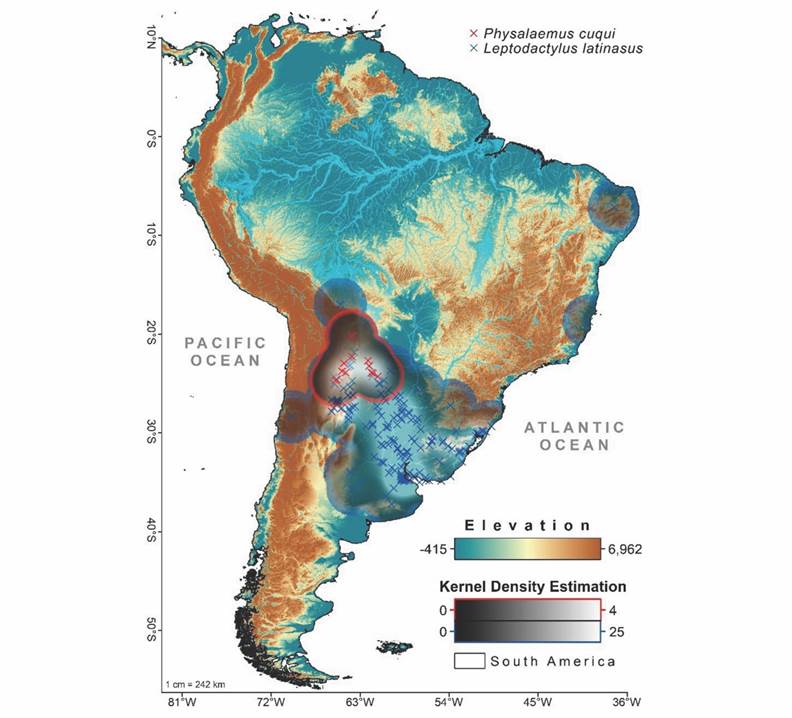
Fig. 1 Digital elevation model with the geographic distribution of Leptodactylus latinasus and Physalaemus cuqui in South America. In grey scale, Kernel density map with areas of highest (white) and lowest (black) abundance density of records of occurrence.
Occurrence records: We selected the Leptodactylus latinasus and Physalaemus cuqui species, from the Leptodactylidae family, because they are sympatric and although both species present similar environmental and climatic requirements, their reproductive modes respond differentially to climatic-environmental conditions. The target species are classified as “Least-concern”: L. latinasus (Lavilla et al., 2004) and P. cuqui (IUCN SSC Amphibian Specialist Group, 2022). Their environmental niches overlap, mainly, with the Chaco region, exposed not only to anthropic environmental simplification but also to the cultural degradation of their traditional communities (De Marzo et al., 2022). For both species, we corroborated the existence of synonymy and possible taxonomic identification errors. In Fig. 2 we summarized the logic and methodology applied in this research, from obtaining the input data to the SDMs evaluation.
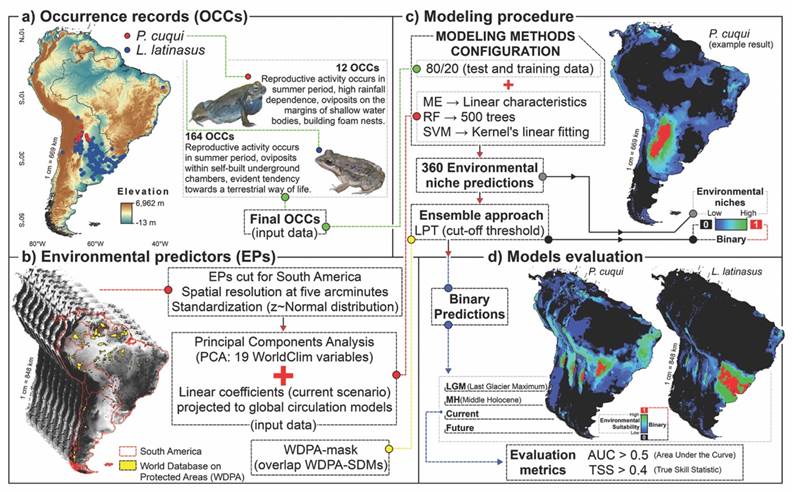
Fig. 2 Logical scheme of the SDMs methodology applied to Leptodactylus latinasus and Physalaemus cuqui in South America. The four main axes are A. Occurrence records (OCCs), B. Environmental predictors (EPs), C. Modeling procedure and D. Models evaluation.
We obtained records of occurrence (OOCs) from speciesLink (speciesLink, 2023a; speciesLink, 2023b) and the Global Biodiversity Information Facility (GBIF) platforms. Although the number of records does not interfere with the algorithm’s performance (De Almeida et al., 2010) we used all the OCCs available in GBIF (L. latinasus (GBIF, 2023a) and P. cuqui (GBIF, 2023b)) without applying filters of sampling methods, sex, size, developmental stage, or specific time period. We removed all OCCs with null or duplicate geospatial data, with errors, or outside the South American geographic boundaries using QGIS software version 3.14 (QGIS Development Team., 2022). The final OCCs for each species totaled 164 for L. latinasus and 12 for P. cuqui (Fig. 2A).
We executed Epanechnikov’s Kernel function, using the ‘Heatmap’ extension of the QGIS software, with 10 000 km of bandwidth (Fig. 1). The Kernel density estimation (KDE) is a non-parametric weighting function that shows the spatial heterogeneity of the occurrence records, indicating areas of greater or lesser sampling effort (Silverman, 1986). We evaluated the spatial autocorrelation (SAC) of the occurrence records with the Moran Index. To reduce possible side effects derived from spatially biased sampling efforts (logistical ease of collection, historical sampling points, precision errors, among others), we used the ‘spThin’ package (Aiello-Lammens et al., 2015). The ‘spThin’ package reduces redundant information; removes occurrence records that could contribute to SAC, increasing SDMs performance and prediction quality (Aiello-Lammens et al., 2015; Guélat & Kéry, 2018). Although both species are sympatric, the OCCs did not show SAC effects (values less than |0.2|), so we did not lose data.
Environmental predictors and protected areas: We used the 19 climate variables from the WorldClim 2.0 platform (Fick & Hijmans, 2017; Hijmans et al., 2005) as environmental predictors (EPs). The selected EPs represent the climatic dynamics for 1) the current climate scenario (1960-1990), 2) the last glacial maximum (LGM: ~22 000 years), 3) the middle-Holocene (MH: ~6 000 years), and 4) the future scenario (2041-2060). We used the CCSM4, MPI-ESM-P, and MIROC-ESM global circulation models due to the high quality and availability of the 19 EPs in all the climate scenarios evaluated (Hijmans et al., 2005; Varela et al., 2015). Combining different climate variables, including greenhouse gas concentration trajectories, global circulation models simulate the atmospheric and oceanic circulation of past and future scenarios (Hijmans et al., 2005; Schwalm et al., 2020). Based on intensified anthropogenic actions and the global context of greenhouse gas emissions (Schwalm et al., 2020), we selected the most extreme greenhouse gas emission scenarios to represent the future scenario (Representative Concentration Paths, RCP = 8.5).
We selected all the EPs with five arcminutes of spatial resolution (~10 km at the equator) since this does not compromise computational capacity and allows a correct spatio-temporal representation of the configuration and environmental dynamics (Barve et al., 2011). This resolution also facilitates the EPs adjustment to possible spatial biases of the occurrence records and reduces the effects of bionomic factors (Guélat & Kéry, 2018; Soberon, 2007). To carry out the EPs standardization (z~Normal distribution: mean of zero and standard deviation of |1|) and avoid unequal weights, we applied the packages ‘maps’ (Becker & Wilks, 1993), ‘rgdal’ (Keitt, 2010) and, ‘raster’ (Hijmans et al., 2015) (Fig. 2B). To incorporate all the potential environmental regions for the target species (Barve et al., 2011), we selected the geographic limits of South America as the modeling area. With a clipping mask with a South American geographic extent and using the ‘raster’ and ‘SDMTools’ packages (Vanderwal et al., 2014), we clipped all EPs with R software (R Core Team, 2019; Fig. 2B). We applied a principal component analysis (PCA) to reduce the multicollinearity effects of the environmental variables (De Marco & Nóbrega, 2018). Before applying the PCA, for each climate scenario, we projected the linear coefficients of the current scenario to the past (MH and LGM) and future climate scenarios. In each temporal scenario, we selected the first eight axes that explained more than 95 % of the original environmental variation in the PEs (Fig. 2B). The presence/absence predictions overlap the (henceforth: binary predictions) between the climate scenarios (LGM-current and current-future) allowed us to estimate the loss, stability, and gain of niches of both species.
To assess the conservation status of species concerning the environmental niches obtained for the current and future scenarios, we superimposed our binary predictions on the protected areas from the World Database on Protected Areas (WDPA) (UNEP-WCMC & IUCN, 2020). To adjust the conservation units to the target species’ ecology and distribution, we selected only protected areas classified as strict reserves of IUCN categories I-IV. We adjusted the spatial resolution and geographic extent of the WDPA mask using the same parameters selected for the EPs. Finally, we used the binary predictions of each climate scenario to calculate the overlap of the environmental niches with the protected areas (Fig. 2B, Fig. 2C).
Species distribution model: Based on the algorithm’s performance and their flexibility concerning the input data (Elith et al., 2006), we applied an ensemble model approach with the Maxent algorithms (Maximum Entropy) (Phillips et al., 2006), Random Forest (RF) (James et al., 2013), and SVM algorithms (Support Vector Machine) (Salcedo-Sanz et al., 2014). This approach allows a better environmental niches approximation, summarizing in a single output matrix the overfitting/underfitting predictions of the algorithms, and representing the maximum/minimum thresholds of environmental suitability (Barve et al., 2011). Starting from the region of lower environmental suitability predicted by the Bioclim algorithm, we geographically delimited the randomly distributed pseudo-absences without spatial coincidence with the OCCs (De Andrade et al., 2020). We selected as a partition method the OCCs division into 80 % to be used as test data and 20 % to be used as training data for the algorithms (Fig. 2C).
Following the parsimony principle, we set the algorithms by default so that the predictions respond to the input variables and not to the parametric settings (Alvarez et al., 2022; De Andrade et al., 2020). In this sense, we configured ME using linear characteristics, and the parameters were selected by default, with one thousand interactions, 10 thousand background points, and logistic type output (Elith et al., 2006). We adjusted the RF settings automatically using 500 trees (Hastie et al., 2009). In the same way, we configured SVM to default using Kernel’s linear fitting function, with probabilistic output (Fig. 2C). Following De Andrade et al. (2020) we ran the different SDMs from the R software using the packages ‘dismo’ (Hijmans et al., 2017), ‘randomForest’ (Liaw & Wiener, 2002) and ‘kernlab’ (Karatzoglou et al., 2004). We defined the randomization process to 30 replicates for each algorithm to reduce potential correlation errors and avoid biased evaluations.
We generated the binary predictions by cutting the environmental niche predictions for each algorithm by the lowest presence threshold (LPT) (Pearson et al., 2007). The obtained binary predictions for each algorithm represent the areas of presence/absence of the target species based on the environmental niche prediction matrices (Barve et al., 2011; Grenouillet et al., 2011). Following the ensemble model approach, we combined and concatenated the binary predictions of all the algorithms in a single output matrix, both for the current scenario and for the past and future projections of both species. For this, we used the weighted average consensus: considering all predictions with true skill statistics (TSS) values above the average TSS value (Grenouillet et al., 2011).
We evaluated the performance of SDMs using the metrics TSS and the Area Under the Receiver-Operator Curve (AUC) (Fielding & Bell, 1997) which attribute different weights to prediction errors (Fig. 2D). The values of TSS and AUC vary between -1 and 1, values greater than 0.5 show that the predictions fit better than the random models (Allouche et al., 2006; Fielding & Bell, 1997). We selected the AUC statistic because, being independent of fixed thresholds, it allows us to assess precision across environmental niches, discriminating areas of omission from areas of known presence (Fielding & Bell, 1997).
Historical niche variation: To calculate the n-dimensional hypervolume variation of environmental niches on a temporal scale, we generated an environmental background within South America using QGIS software. This selection allowed us to consider regions with suitable environmental conditions for the species and minimize the inclusion of areas where they might not be found due to the presence of physical barriers or biotic interactions (Kubiak, et al., 2017). We generated the environmental background by randomly distributing 1 000 points for each scenario (past, current, and future). We used the minimum-volume ellipsoids to visualize the ecological niches in a three-dimensional space built from the first three principal components and with a convex polyhedron (Qiao et al., 2016; Soberon & Nakamura, 2009; Van Aelst & Rousseeuw, 2009). We conducted the analyses with NicheA, a software for exploring and analyzing the environmental and geographic spaces of both virtual and real species (Qiao et al., 2016). These analyses calculate the overlap of environmental niches and allow us to evaluate possible past and future variations concerning the current configuration of ecological niches (Qiao et al., 2016).
Results
Occurrence records and model evaluation: The KDE evidence niche overlap and spatial heterogeneity in sampling efforts (Fig. 1). After applying the spatial filter from the ‘spThin’ package, we possibly reduce the residual effects of the SAC on the OCCs, increasing the overall performance of all applied algorithms. In fact, all the algorithms showed good performance (SMG 1) with high mean values in the statistics evaluated for L. latinasus (TSS = 0.84 ± 0.04, AUC = 0.94 ± 0.02 (mean ± standard deviation)) and for P. cuqui (TSS = 0.90 ± 0.12, AUC = 0.96 ± 0.05). Although all the algorithms had high performance, SVM obtained the highest values of both AUC and TSS.
Environmental niches and binary predictions: To identify environmental niches and presence/absence areas, we visually combined the potential environmental suitability with the binary predictions of each temporal scenario and for each species (Fig. 3). The environmental niches showed different spatial complexities and connectivity between both species in each climatic scenario. However, despite the differences in the spatio-temporal distribution patterns, both species show geographic fidelity associated with the central region of their historical distribution (Fig. 3).
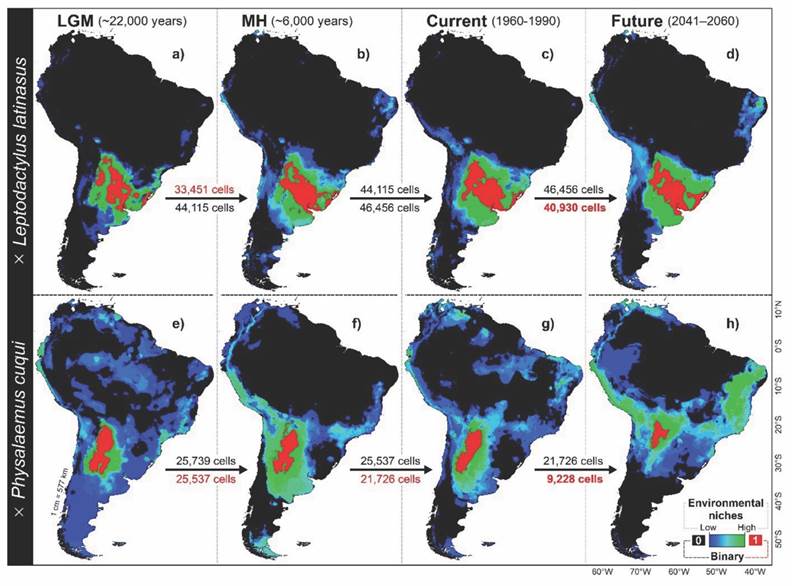
Fig. 3 Spatio-temporal distribution patterns obtained for the species Leptodactylus latinasus (A., B., C. and D.) and Physalaemus cuqui (E., F., G. and H.) in different temporal settings. Potential environmental niches on color scales (blue and green) and binary predictions with absence (black color) and presence (red color).
The environmental niches of L. latinasus were geographically delimited between the parallels 20 °S and 40 °S of South America, more precisely to the low altitude region of southern Bolivia, southeastern Paraguay and Brazil, central Argentina, and northern from Uruguay. In the LGM (Fig. 3A) and MH scenarios (Fig. 3B), the environmental suitability predictions of L. latinasus suggest aggregate distribution patterns of low spatial complexity and high connectivity between patches. While the binary predictions were restricted to the alluvial plains of central Argentina and Uruguay, which, connected by a “diagonal axis”, constitute the reported distribution center for both species. During the LGM scenario, approximately 33 451 cells (3.4 % of the total) were predicted for L. latinasus, and the number of cells increased to 44 115 (4.5 % of the total) in the MH scenario (Fig. 3). The environmental niche of L. latinasus in the current scenario (Fig. 3C) experienced a second increase, reaching 46 456 cells (4.7 % of the total) and showing distribution patterns with high spatial connectivity and low complexity. The diagonal axis that joins Argentina-Uruguay could be maintained in the future scenario (Fig. 3D) with a more complex spatial pattern and less connectivity between the patches, especially in the central region. In fact, with 40 930 cells (4.1 % of the total) in the current-future transition, predictions for L. latinasus suggest that this species could decrease the width of its environmental niche (Fig. 4B).
For P. cuqui, the environmental niches of the LGM scenario, with 25 739 cells (2.6 % of the total), suggest a high spatial complexity: diffuse and disconnected patches (Fig. 3E). However, the binary predictions of the LGM predict the presence of environmental niches along a North-South axis that perhaps crossed the central region of Argentina latitudinally (Fig. 3E). Although P. cuqui maintained its distribution in the North-South axis during the MH, it could have experienced environmental niche fragmentation and reduction, with isolated patches totaling 25 537 cells (2.5 % of the total). For the current (Fig. 3G) and future (Fig. 3H) scenarios, P. cuqui continues to experience fragmentation and loss of environmental niches. In fact, for the current scenario, with 21 726 cells (2.2 % of the total), the predictions of environmental suitability of P. cuqui show high spatial complexity with isolated patches that increase the fragmentation and vulnerability of their populations. However, unlike other scenarios, the binary predictions showed few isolated patches, with high spatial connection and continuity along the North-South axis. In the future scenario, binary predictions suggest that P. cuqui would experience the greatest loss of its environmental niches, decreasing to 9 228 cells (0.9 % of the total). In this sense, climate change will reduce the environmental niches of P. cuqui by 42.5 %; this spatial retraction represents 12 498 cells (57.5 % of the total).
Temporal scenarios and protected areas: The visualization of the environmental niche using the n-dimensional hypervolume showed that the current environmental requirements of L. latinasus have not varied considerably about the LGM (SMG 2A, SMG 2B). Similarly, from the minimum-volume ellipsoids, some similarity is observed when comparing current environmental conditions with future ones, suggesting stability and environmental fidelity (SMG 2C). In contrast, the n-dimensional hypervolume of P. cuqui showed historical fluctuations between past and current environmental requirements, mainly evident during the MH scenario (SMG 2D, SMG 2E). Although the loss of the environmental niche is progressive and continuous from the LGM to the current (Fig. 3), the minimum-volume ellipsoids confirm environmental niche loss for P. cuqui when comparing current and future scenarios (SMG 2F).
The environmental niches in both species’ current and future scenarios (Fig. 4) show high overlap in the minimum-volume ellipsoids (Niche spatial overlap = 31 %). This overlap increased considerably in the future scenario (niche spatial overlap = 70 %), possibly due to the increase in environmental sympatry between both species derived from the environmental niche reduction of P. cuqui (Fig. 5).
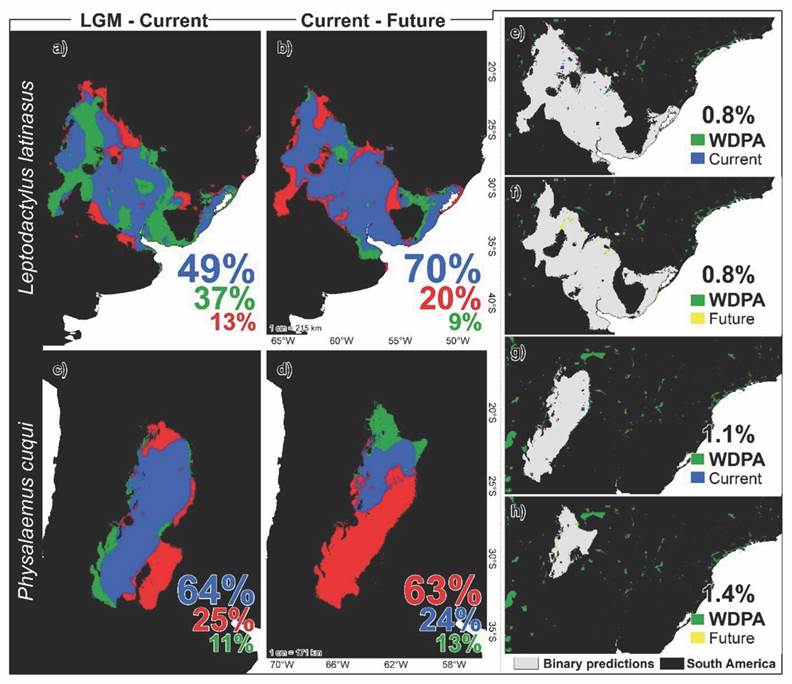
Fig. 4 Spatio-temporal distribution of lost (red), gained (green), and stable (blue) environmental niches in the LGM-current and present-future transitions for Leptodactylus latinasus (A. and B.) and Physalaemus cuqui (C. and D.). Spatial overlap of environmental niches in the current and future scenarios for L. latinasus (E. and F.) and P. cuqui (G. and H.) in relation to the WDPA conservation units.
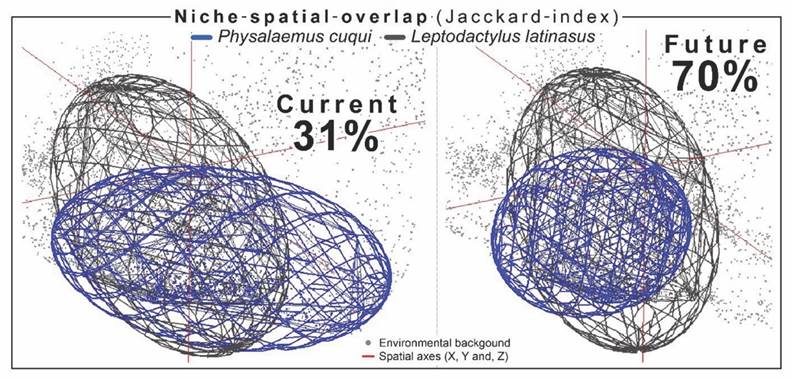
Fig. 5 Spatial overlap of the environmental niches obtained for Leptodactylus latinasus and Physalaemus cuqui in current and future scenarios. The n-dimensional hypervolume of the environmental niches, defined by the environmental variables (background points, gray color), is represented by the ellipsoids in the spatial axes (red lines) and responds to the Jacckard index.
For both species, the temporal transitions LGM-current and current-future suggest different proportions of lost, gained, and stable niches (Fig. 4). Temporal transitions of L. latinasus showed 49 % stable niches in the LGM-current scenario and 70 % in the current-future scenario, suggesting high spatio-temporal fidelity in these environmental regions (Fig. 4A, Fig. 4B). In the LGM-current transition (Fig. 4A), L. latinasus lost 13 % of its niches, while in the current-future transition it would lose 20 % (Fig. 4B). On the contrary, for P. cuqui, all the temporal scenarios were negative; in all temporal transitions, the species suffered fragmentation and loss of environmental niches (Fig. 4C). In the LGM-current transition P. cuqui loses 25 % of its environmental niches, while in the current-future transition, it loses 63 % (Fig. 4D).
The binary predictions overlap with the WDPA-protected areas shows that these areas cover a small proportion of the environmental niches of both species, both in the current and future scenarios (Fig. 4). For L. latinasus the predictions obtained for the current and future scenarios show 0.8 % overlap with protected areas (Fig. 4E, Fig. 4F). For P. cuqui the spatial overlap of its predictions for the current scenario was 1.1 % (Fig. 4G) and for the future, it was 1.4 % (Fig. 4H).
Discussion
The KDE function visually represented the geographic distribution and the sampling efforts, identifying subsampled areas of ecological interest for these species and their critical niches (Hortal et al., 2015). The high performances obtained could derive from the input data pre-processing; the reduction of the SAC effects on the occurrence records and the multicollinearity decrease of the predictors (Fig. 3). Even so, since the AUC statistic is conditioned by the calibration area and represents a source of overfitting, we complement the performance evaluation with the TSS statistic that depends on the input data quality (Allouche et al., 2006; Leroy et al., 2018). In the LGM-current scenario, the predictions suggest that L. latinasus maintained high environmental fidelity (49 %), with 37 % expansion and 13 % loss of environmental niches (Fig. 4, SMG 2). L. latinasus could be coupled to climatic variations: 70 % of the stable niches suggest high climatic-environmental fidelity in the current-future transition. However, L. latinasus loses more niches than it could gain in a climate change scenario, suggesting restricted distributions and a tendency towards microendemism. We estimated that from the LGM to the current scenario, P. cuqui gained stable environmental niches (64 %), perhaps due to the environmental expansion of its populations (Fig. 4, SMG 2). Climate change would reduce 40 % of the stable environmental niches of P. cuqui, forcing their populations to move northward (Fig. 4, SMG 2). Regardless of spatial sympatry or reproductive modes, climate change could promote microendemism and the extinction of species currently classified as “Least-concern”, with stable populations (Burger et al., 2019; IUCN SSC Amphibian Specialist Group, 2022; Lavilla et al., 2004). Spatial distribution patterns and their temporal fluctuations vary; therefore, it is important to consider the different temporal scenarios to manage and apply appropriate conservation actions to each species (Alvarez et al., 2021; Alvarez et al., 2022).
The predictions obtained for the current scenario allow us to identify environmental niche regions not only for these species but also for other species of the family (Medina et al., 2020; Soberon, 2007). These results facilitate the objective planning of future samplings and allow for defining management and mitigation actions in regions exposed to intensified anthropic actions in agricultural deforestation and mining exploitation (De Marzo et al., 2022). Consistent with our predictions, the family Leptodactylidae has been reported from the southern tip of Texas (United States), Southern Sonora (Mexico), and the northern Antilles to southern regions of Brazil, Argentina, and Chile (Frost, 2022; Medina et al., 2020). For L. latinasus we estimate wide environmental niches and, it is expected that in the face of future climate change, the species will maintain the proportion of potential environmental niches. This could be adjusted to the high species’ environmental plasticity and the ability to adapt to ecosystems with different levels of anthropic intervention (Ponssa & Barrionuevo, 2008). Binary predictions for P. cuqui showed progressive declines in spatial distributions (Fig. 3), maintaining spatial fidelity along an imaginary north-south axis in the Argentine lowlands. For the Yungas Biome, in the northern portion of Argentina, it was estimated that P. cuqui would lose more than 50 % of its environmental niches because of its specificity and selectivity of habitats (Andrade-Díaz et al., 2021). Although in the LGM-current transition, the number of stable niches is high, in the current-future transition the sum between the niches gained and stable does not exceed the number of niches lost by P. cuqui. Indeed, we predicted losses in environmental niches for the HM scenario, but the largest niche losses for this species were predicted for the future scenario (Fig. 4, SMG 2). This suggests that climate fluctuations, such as those reported during the HM (Kohler et al., 2010; Sobral-Souza et al., 2015) and those predicted for the future (Bellard et al., 2012; Medina et al., 2020; Menéndez-Guerrero et al., 2020), determined and define the spatial and demographic patterns of these species (Andrade-Díaz et al., 2021; Blaustein et al., 2010; Carey & Alexander, 2003).
Three points to highlight in the spatio-temporal distribution patterns of the predicted environmental niches: first, in the current-future transition, the availability of environmental niches decreases. Second, the environmental sympatry between both species increases, possibly due to the decrease in the environmental niche of P. cuqui (Fig. 5). Third, the spatial predictions were associated with lowland regions of central Argentina (Fig. 4). Menéndez-Guerrero et al., (2020) suggest that the regional topography is a deterministic variable of changes in the anurans α and β diversities. Tropical mountainous areas harbor high anuran biodiversity with restricted distributions: rugged topography favors local endemism (Anderson et al., 2011; Magurran et al., 2019; Menéndez-Guerrero et al., 2020). In contrast, the lowlands are inhabited by generalist species, with wide spatial distributions since the regional topography does not represent physical barriers to the dispersal and accumulation of water (Menéndez-Guerrero et al., 2020). In this sense, higher altitude regions favor a decrease in β diversity and an increase in α diversity, while lower altitude regions respond oppositely (Anderson et al., 2011; Magurran et al., 2019; Menéndez-Guerrero et al., 2020). Our target species could respond to these landscape characteristics, associating with lowlands with historical climatic and hydrological stability (Andrade-Díaz et al., 2021; Menéndez-Guerrero et al., 2020). Climate change in synergy with anthropic actions could cause alarming declines not only in the environmental niches of these species but also in anuran biodiversity and their critical habitats (Alvarez et al., 2021; Alvarez et al., 2022; Exbrayat, 2018; Menéndez-Guerrero et al., 2020).
In the current-future transition, both species lose environmental niches, in parallel, the percentages of areas designated for their protection also decrease. According to the IUCN, both L. latinasus and P. cuqui (IUCN SSC Amphibian Specialist Group, 2022; Lavilla et al., 2004) are classified as “Least-concern”. However, the environmental niches for both species show a low spatial overlap with areas designated for conservation in the current and future scenario, where climate change would act as an aggravating factor (Fig. 4). Despite deterministic climate change effects, the greatest risk lies in the conservation units: few, small and disconnected. In the current and future scenarios L. latinasus (with 70 % stable niches), conserves 0.8 % of its niches, while P. cuqui (with 63 % lost niches) conserves less than 2 % of its environmental niches (Fig. 4E, Fig. 4F). The accumulated and intensified effects of climate change will decrease water availability in the coming decades, conditioning the spatio-temporal distribution patterns of anurans (Medina et al., 2020). Global warming and variations in rainfall regimes and hydrological networks limit the reproductive behaviors and oviposition of different species of anurans (Blaustein et al., 2010; Bruzzi-Lion et al., 2019; Clavel et al., 2011; Martins, 1988; Pounds et al., 2006). This added to the regionally intensified land use, could modify the spatial and demographic patterns of currently stable populations (De Marzo et al., 2022). The reproductive modes of anurans could emerge as determinants of their reproductive success; for most species, reproduction occurs during the warm season, coinciding with the rainy season (Exbrayat, 2018).
With spatial hierarchy effect, global climatic variables define the regional environmental conditions and local biotic interactions, establishing the environmental niches of the species (Domisch et al., 2015). In this sense, spatial sympatry partially responds to climatic and environmental characteristics of shared niches: both species couple their reproductive activities and synchronize oviposition with the rainy season (Ferrari & Vaira, 2001; Iglesias & Natale, 2013; Ponssa & Barrionuevo, 2008). L. latinasus, like other species of the genus, initiates courtship activities and the reproductive period even when the rains are not abundant and when temporary bodies of water are dry (Downie & Smith, 2003; Martins, 1988; Ponssa & Barrionuevo, 2008). In turn, L. latinasus oviposits inside foam nests that it builds in underground chambers; this thermal insulation increases the chances of reproductive success (Martins, 1988, Ponssa & Barrionuevo, 2008). The underground chambers and the foam prevent larvae dehydration in case of late rains and severe dry conditions (Downie & Smith, 2003; Ponssa & Barrionuevo, 2008). With the rain, the larvae hatch inside the chamber and reach the bodies of water to complete their development, showing tendencies towards terrestrial life forms (Martins, 1988; Ponssa & Barrionuevo, 2008). The environmental and behavioral plasticity of L. latinasus would partially argue the stability/width of its historical environmental niches, explaining its better performance against climate change (Bardier et al., 2014). P. cuqui depends on frequent and intense rains, forming vocal choruses at the beginning of the rainy season, from inside water bodies of variable depth and with margins covered by herbaceous vegetation (Ferrari & Vaira, 2001; Iglesias & Natale, 2013). The oviposition of P. cuqui has a pattern similar to that of other species of the genus that build foam nests on the margins of water bodies (Ferrari & Vaira, 2001; Acosta’s observation). The specificity/selectivity of P. cuqui for niches with specific climatic, edaphic, and vegetation conditions restrict the amplitudes of its environmental niches. There are different knowledge gaps not only about the reproductive modes of anurans but also about the performance of these modes against the effects of climate change. Abrupt climatic variations could lead habitat specialist species, such as P. cuqui, to lose demographic and biogeographic stability, increasing their vulnerability to extinction (Andrade-Díaz et al., 2021; Burger et al., 2019).
The target species are currently not at risk of extinction and, their populations are probably stable, but climatic conditions resulting from climate change suggest that their environmental niches will decline, following the fate of other anurans (Andrade-Díaz et al., 2021; Burger et al., 2019; Menéndez-Guerrero et al., 2020; Pounds et al., 2006; Stuart et al., 2004). Extreme drought events coupled with hydrological variations could compromise their physiological tolerance thresholds and decrease their reproductive performances (Hijmans et al., 2005; Menéndez-Guerrero et al., 2020). Our results suggest that the loss of anuran environmental niches could increase environmental sympatry, pressuring spatial responses coupled with competition; new ecophysiological barriers derived from climatic and anthropic barriers (Bruzzi-Lion et al., 2019; Exbrayat, 2018). In this sense, since the period of reproductive activity of anurans is associated with seasonality, changes in climatic and environmental dynamics could modify the reproductive activities of these species (Medina et al., 2020). The predictions identify stable historical niches; From the LGM to the current scenario, environmental niches are showing where species conservation actions should be focused.
Ethical statement: the authors declare that they all agree with this publication and made significant contributions; that there is no conflict of interest of any kind; and that we followed all pertinent ethical and legal procedures and requirements. All financial sources are fully and clearly stated in the acknowledgments section. A signed document has been filed in the journal archives.
See supplementary material