Introduction
New Scientific knowledge and techniques in remote sensing (RS) have been developed because of the interest in understanding spatial behaviors of different phenomena, such as land use, natural resources exploration and exploitation, and natural hazards. The volcanic activity is one of the phenomena that highly influence the development of the Earth’s crust but also affects the bio and atmosphere. In general, a volcanic eruption is an event of short duration that can be measured (Araña & López, 1974) and it is of research interest because of its seismic activity, chemical contribution to the atmosphere, and effects on landslides, fauna and vegetation. Often, volcanoes negatively impact people, urban infrastructure, and nature. RS is especially important because it allows to monitor this type of natural activities by sensing the behavior of the different environmental factors. Also, RS is useful for predicting, monitoring, mapping, and management of the volcanic risk (García & Dávila, 2014).
Costa Rica has a high volcanic presence with 62 main volcanic vents and systems (Alvarado, 2008) . This is the case for the Turrialba volcano, which presented a rise in volcanic unrest since 2013 (RSN, 2017) that caused evident damage to the surrounding vegetation. However, the spatiotemporal behavior of this damage since 2013 had not been evaluated yet. Satellite images are suitable to carry out such an assessment because they have some advantages like: non-destructive observation, wide range spectrum information and periodic coverage (Chuvieco, 2010). Thus, RS techniques can be used to determine the evolution of the damage caused by volcanoes and other natural events, which have spatiotemporal variations.
Some RS techniques are specially used to map the phenology -cyclic and seasonal natural phenomena of vegetation and fauna- of the vegetation such as Normalized Difference Vegetation Index (NDVI) and Land Surface Temperature (LTS), which have been used for landcover change analysis (Escuin, Navarro, & Fernandez, 2008; Sobrino & Raissouni, 2000). Also, the NDVI has been used to analyze desertification (Tucker, Dregne, & Newcomb, 1991), which is a similar process in terms of vegetation loss for the surrounding areas of the Turrialba volcano because of the volcanic activity. Moreover, the Normalized Burn Ratio (NBR) is another vegetation index used to determined burned areas, which is less used because of the lack of the mid-infrared band in some older sensors (Escuin et al., 2008) and can be useful to contrast the affected areas.
Tortini, van Manen, Parkes and Carn (2017) performed this analysis for the Turrialba volcano using the Enhanced Vegetation Index (EVI) derived from Aster images. Results showed the evolution of the vegetation loss and contrasted it with ground sampling to determine acid deposition since 2005 until 2013. Although there was an increase of the vegetation loss rate from 2007 to 2010, this was stabilized after that period (Tortini, van Manen, Parkes, & Carn, 2017). Furthermore, not only Aster but also Landsat were recommended to carry out this kind of studies. The U.S. Geological Survey, with the Landsat program, provides free and high quality multispectral imagery that has been acquired for more than 4 decades (U.S. Geological Survey, 2017). Also, the new program Landsat 8 provides higher radiometric and spectral resolution.
The objectives of this work are to evaluate the use of the vegetation index, NDVI and NBR, for spatial-temporal analysis to determine the vegetation loss in the surrounding areas of the Turrialba volcano from 2013 to 2017, and to contrast the area determination from the two techniques. Also, we extend the previous work done by Tortini et al. (2017) by comparing their results with those presented in this article. First, the data and methods are described to provide the proper background. Second, the results of the spatial temporal analysis are shown for the years of 2014, 2016 and 2017. Finally, conclusions are made based on the behavior of the indices and the future work is discussed.
Data and methods
Study Area and Period
The Turrialba volcano is located 24 km northwest from the Turrialba Town in Cartago, Costa Rica, and is part of the Central Volcanic Range (Alvarado, 2008). The study area corresponds to 21.7 km2 in the surrounding areas of the Turrialba volcano crater (Fig. 1). The chosen period is from 2014 to 2017, which matches with the beginning of the high volcanic activity cycle (RSN, 2017) and the end of the study made by Tortini et al. (2017). Moreover, the dry season was chosen because there is more possibility to acquire images with less percentage of cloud coverage and the vegetation is influenced by the same seasonal conditions. The dry season in this region, occurs from December to May.
Landsat 8 Images
The Landsat 8 satellite was launched on February 11, 2013, and carries a sensor for mapping the Visible Light Spectrum (VIS) and the thermal spectrum: Near Infrared (NIR), Short Wave Infrared (SWIR), and Thermal Infrared (TIR) (U.S. Geological Survey, 2015). The Landsat 8 sensors generate images with a spatial resolution of 30 m and 16 bits radiometric resolution. This imagery is referred to the World Reference System (WRS) as a global reference notation for cataloging. The WRS uses a notation of path and row for a determined image and each image covers a surface area of 190-by-180 km (U.S. Geological Survey, 2016); The image that covers Costa Rica’s center is described by path and row 15 and 53 respectively. Table 1 shows the ID, month and year of the images used in this project.
Table 1 Information of the images used in this project.
ID | Month | Year |
---|---|---|
LC08_L1TP_015053_20140219_20170307_01_T1 | February | 2014 |
LC08_L1TP_015053_20160108_20170224_01_T1 | January | 2016 |
LC08_L1TP_015053_20160413_20170223_01_T1 | April | 2016 |
LC08_L1TP_015053_20170211_20170228_01_T1 | February | 2017 |
LC08_L1TP_015053_20170211_20170228_01_T1 | February | 2017 |
Normalized Difference Vegetation Index (NDVI) and Normalized Burned Ratio (NBR)
The NDVI is an index which assesses the light reflected by the plants in the NIR and Red band range. These two bands show the main response to the presence of vegetation due to the presence of chlorophyll: with a higher chlorophyll content, the absorption of the Red band increases resulting in a higher foliar volume and a larger NIR band reflection (Gonzaga, 2014). Figure 2 shows this phenomenon graphically. The NDVI is defined as the ratio of the Red and NIR bands difference with respect to the summation of this two bands (Escuin et al., 2008; Sobrino & Raissouni, 2000). Equation 1 shows this ratio. This index produces values from -1 to 1; theoretically, the 0.2 value is a threshold number between soil and vegetation, where values above the threshold number indicate the presence of vegetation and a value less or equal shows the presence of bare soils (Sobrino & Raissouni, 2000).
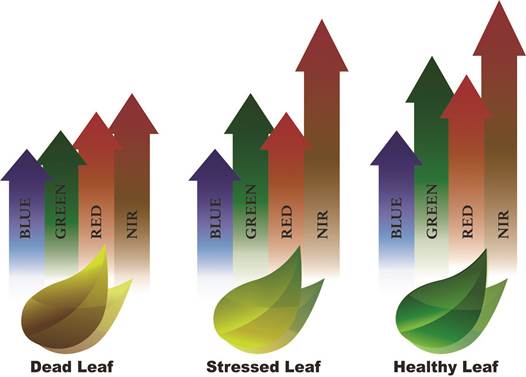
Fig. 2: Band reflectance behavior for different state of leaf health. Source: Mckinnon (2016), modified for this study.
The NBR is used to detect burned vegetation and defined as the ratio of the difference between the NIR and the Short-Wave Infrared (SWIR) bands and the sum of these two bands, as described by equation 2. This index, as the NDVI, results in values ranging from -1 to 1. However, for the NBR index, the threshold value is 0. Thus, positive values indicate the presence of vegetation and negative values are interpreted as burned vegetation (Escuin et al., 2008). A similar behavior of the affected vegetation around Turrialba volcano was assumed as a product of the volcanic activity.
The Landsat 8 bands used are: Band 4 (Red), Band 5 (NIR) and Band 6 (SWIR1). The spectrum range values of these three bands are shown in Table 2. A more detailed description of the bands can be found in the Landsat 8 (L8) Data User Handbook (U.S. Geological Survey, 2016).
Image Processing
Filtering
The process of filtering can be time consuming because there is a high cloud coverage in a tropical country like Costa Rica. For example, an image could have a low percentage of cloud coverage but this coverage could be consistently located through time in mountainous areas such as Turrialba volcano. Because of this situation, the following two processes of cloud assessment have to be performed: a general filter using the cloud percentage number of the image provided by Landsat, and a detailed assessment of the study area. The cut-off value for the general assessment was 40% of cloud cover for the complete Landsat 8 tile. Then, the study area was assessed independently from the cloud assessment of the whole image. Preferably, cloud free images are requested for these studies. If no cloud free images are available for the area and period, several images are combined in a limited time frame in order to create a composite with the lowest cloud coverage possible. The cloud assessment was done using the QGis plugin Cloud Masking with the Fmask algorithm (Corredor Llano, 2017). This algorithm creates masks in the presence of clouds and snow (Zhu & Woodcock, 2012) and it was recently improved to work with Landsat 8 images (Zhu, Wang, & Woodcock, 2015). Some masks are shown as an example in Figure 3; the white color shows cloud presence and the black color represents clear sky.
Correction and Image Combination
The correction of the image for the presence of clouds was done using the cloud masks and raster calculator from QGis. A mask containing 0 forclouds and 1 for clear sky was multiplied by the chosen base image, which had the lowest cloud content, this operation produced a cloud filtered image. Moreover, a mask containing a value of 1 for clouds was multiplied by another Landsat 8 image and the product of this operation produced a filling image which was added to the base image, producing a corrected raster. Figure 4 shows the flow chart of this process. Also, a radiometric correction is performed to minimize the problem caused by different light conditions between images. This process is briefly described in the following paragraphs but further information on radiometric correction is provided by U.S. Geological Survey (2016).
The 16 bit images provide digital number (DN) values from 0 to 216 (65535). A radiometric correction must be applied to compute top of atmosphere (TOA) reflectance values, which is a unitless quantity that accounts for the position of the sun.
The two-step process for computing TOA values is shown in equations 3 and 4. By obtaining TOA reflectance values, correction models can be applied to reduce the atmospheric scattering effect. The Dark Object Subtraction (DOS) model is applied for this study. More information about this correction is given by Chavez (1996).
Where:
ρλ' is the TOA reflectance values corrected by the sun position (unitless);
Mρ is a reflectance multiplicative factor for the specific band;
AL is a reflectance additive factor factor for the specific band;
Qcal is the L1 product DN value.
Where:
ρλ' is the TOA reflectance values corrected by the sun position (unitless); θ is the sun elevation angle
All these parameters can be found in the metadata file (MLT) for each Landsat 8 image.
Area Calculation
The NBR and NDVI values are computed once the process of filtering and correction has finished. The counting box method was used to measure the area; NDVI values lower than 0.2 and negative NBR values were counted as bare soil and dead vegetation. Finally, the pixel count was multiplied by the spatial resolution of the image (900 m2) in order to get the final surface values. Figure 5 shows the summarized process followed in this work.
Results and discussion
Cloud free images were available for February 2014 and 2017 but not for 2015 and 2016. In the case of 2015, the cloud coverage could not be corrected because none of the images for this year had enough cloud free surface. For the year 2016, images for January and April were combined to create cloud free images. This process generated useful information besides the implications such as slightly different values between images for phenology and light variations. As seen in figure 6, there is no considerable change between the 2014 NDVI image and 2016 image. On the contrary, there is a sharp decrease of the NDVI values for 2017. Correspondingly, there is similar behavior of the NBR shown in Figure 7 for the years 2014 and 2016 and there is a decrease in the NBR values for the year 2017. Also, most of the changes are happening toward the west side of the volcano.
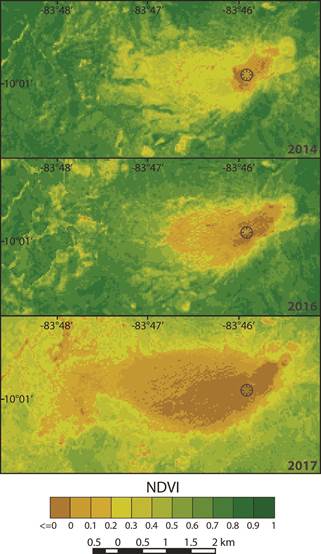
Fig. 6: NDVI images generated with Landsat 8 for years 2014, 2016, and 2017. The affected zone with NDVI values lower than 0.2 is shown in brown colors. Coordinates are shown in WGS84.
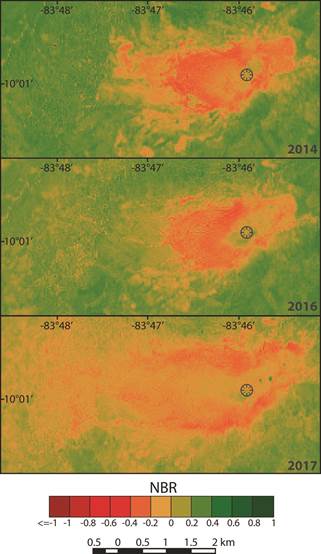
Fig. 7: NBR images generated with Landsat 8 for years 2014, 2016, and 2017. The affected zone with NBR values lower than 0 is shown in orange/red colors. Coordinates are shown in WGS84.
Table 3 shows the surface covered by vegetation and bare soil calculated for the Landsat 8 images in Figures 6 and 7. The affected area for 2017 increased to 7 km2, which corresponds to a 33% of the study area. If the rate of change is considered from 2016 to 2017, the increase in the surface of bare soil is 400% higher than the one observed for the period from 2014 to 2016.
Table 3 Land cover and surface by year from NDVI and NBR indices.
Index | Year | Vegetation (km 2 ) | Affected Vegetation and soils (km 2 ) |
---|---|---|---|
NDVI | 2014 2016 | 21.03 19.77 | 0.64 1.90 |
- | 2017 | 14.61 | 7.06 |
NBR | 2014 2016 | 18.12 19.17 | 3.55 2.50 |
- | 2017 | 14.47 | 7.20 |
Total Studied Area 21.67 km2 |
The histograms shown in Figure 8 describe how the observed NDVI value behaves with respect to the theoretical value of 0.2, which is the threshold between bare soil and vegetation. There is not a significant change during the 20142015 period as suggested by Figure 6. Likewise, the NBR histograms shown in Figure 9 presented the same tendency but with respect to the 0 value limit, which is the threshold between healthy and burned vegetation. Both assessments, spatial and histogram analysis, show a considerable change towards the corresponding values for soils and dead vegetation. Thus, this change is consistent between indices and a good estimate of the volcano’s effect on the vegetation.
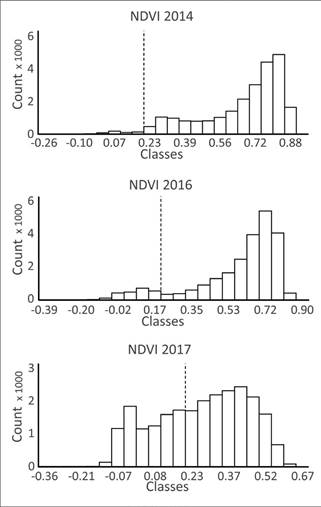
Fig. 8: NDVI histograms for Turrialba Volcano. The stippled lines show the 0.2 theoretical limit for soils vs. vegetation. Columns to the left of the 0.2 value line indicate presence of bare soil, columns to the right of the line indicate presence of live vegetation.
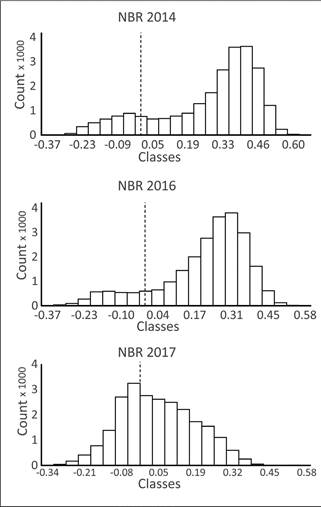
Fig. 9 NBR histograms for Turrialba Volcano. The stippled lines show the 0 theoretical limit for burned vs. live vegetation. Columns to the left of the 0 value line indicate the presence of burned vegetation, columns to the right indicate presence of live vegetation.
Figure 10 summarizes the tendency of the area around the volcano. The NDVI values show an increase in area with values lower than the theoretical limit of 0.2. Also, a higher rate of change can be seen for the 2016-2017 period. Although the NBR tendency behaves differently than the NDVI for 2014 and 2015, they are similar for 2016 and 2017 years.
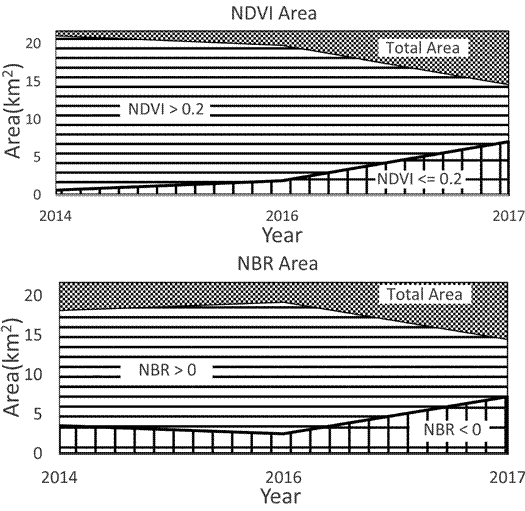
Fig. 10: Surface coverage for both indices (NDVI and NBR). The lower area represents affected vegetation and soils; the middle area represents healthy vegetation; the upper area represent the total surface.
Thus, both indices are consistent in showing a significant change from 2016 to 2017. Tortini et al. (2017) also showed an increment of the affected area from 2007 to 2010 stabilizing after that. These researchers argue that this increment might be due to the change in SO2 but prior might be due to diffuse CO2 degassing.
Because the activity of the Turrialba volcano is ongoing, there is still room for more studies in this field. For further studies, we recommend to focus more on the 2008-2010 and 2016-2017 period in order to better explain the causes of the increase in affected area.
Conclusions
Landsat 8 satellite images were used to determine the areas affected by the ongoing activity of Turrialba volcano eruptions in terms of decrease in vegetation coverage overall, and change in vegetation health. The study area consisted of 21.7 km2 and the study period was from 2014 to 2017. First, images were radiometrically corrected and cloud assessed. High quality images were found for years 2014 and 2017 in the studied area but none for 2015 and 2016. A reconstruction of an image could to be done for 2016, which proved to be of great value to better describe the temporal phenomenon. The NDVI and NBR show a slightly different tendency of the values for the 20142016 period. However, a similar increment of the area towards the soil and dead vegetation was shown for the 2016-2017 period. This situation was determined from the spatial and histogram analysis. A similar increase in the rate of vegetation was shown by Tortini et al (2017), where the authors argue that it could be a product of exposure of the vegetation to SO2 and CO2. For further studies, more research should be done focussed on the 2008-2010 and 2016-2017 period. On the other hand, we are currently working on an automated online service which provides historical assessments of satellite images in volcanic areas of Costa Rica. We believe that this tool will provide useful information to researchers in different fields. Finally, remote sensing (RS) proved to be a very important and useful tool for monitoring local volcanic activity such as the one presented in this article.